MIT study: AI models fail to replicate human responses
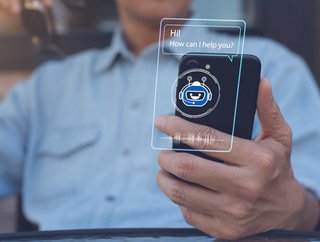
A study conducted by MIT has revealed that AI models have failed to reproduce human judgements about rule violations. According to researchers, AI models trained using common data-collection techniques prove to judge violations more harshly than humans would. In a time where governments and industries are considering greater use of AI and machine learning systems, MIT have deemed that it may be useful to consider if the systems can reproduce human judgement.
The paper, published in Science Advances, details how ‘descriptive data’ has been used to train machine-learning models that judge rule violations, the result of which being that the models tend to over-predict the rule violations. Data that is labelled descriptively typically means that humans are asked to identify factual features (ie. a particular aspect of a photo).
Descriptive data: issues with misclassification
MIT researchers surmised that being less accurate could have serious real-world consequences. This is particularly the case with its harsher judgements, with MIT using the example of a descriptive model being used to make decisions about if an individual is likely to reoffend. They suggest that this could lead to higher bail amounts, or even longer criminal sentences.
Findings also demonstrated that if descriptive data was used to train a model, it will underperform a model trained using normative data. The descriptive model in particular is more likely to misclassify inputs by falsely predicting a rule violation.
Marzyeh Ghassemi, an assistant professor and head of the Healthy ML Group in the Computer Science and Artificial Intelligence Laboratory (CSAIL), said; “I think most artificial intelligence/machine-learning researchers assume that the human judgements in data and labels are biased, but this result is saying something worse.”
“These models are not even reproducing already-biased human judgments because the data they’re being trained on has a flaw: Humans would label the features of images and text differently if they knew those features would be used for a judgement. This has huge ramifications for machine learning systems in human processes.”
This ultimately raises further questions concerning linguistic bias that AI, and chatbots in particular, are susceptible to. AI Magazine recently reported that the bias is so ingrained that even AI chatbots have it built in.
“This shows that the data do really matter. It is important to match the training context to the deployment context if you are training models to detect if a rule has been violated,” lead author Aparna Balagopalan said to MIT News.
Extensive research has been undertaken into AI language models prior to the MIT study, with GPT-3 in particular having been proven to be comparable to humans after psychological testing in deliberation and causal reasoning. However, it is clear that there are discrepancies between humans and AI that cannot be ignored.
As part of a study published in April 2023, entitled ‘More is Better: English Language Statistics are Biased Toward Addition,’ researchers at the University of Birmingham asked GPT-3, the predecessor of ChatGPT, what it thought of the word ‘add’.
It replied: “The word ‘add’ is a positive word. Adding something to something else usually makes it better. For example, if you add sugar to your coffee, it will probably taste better. If you add a new friend to your life, you will probably be happier.”
AI-users must be aware of linguistic bias
Dr Bodo Winter, Associate Professor in Cognitive Linguistics at the University of Birmingham, commented on the subject: “The positive addition bias in the English language is something we should all be aware of. It can influence our decisions and mean we are predisposed to add more layers, more levels, more things when in fact we might actually benefit from removing or simplifying.
“Maybe next time we are asked at work, or in life, to come up with suggestions on how to make improvements, we should take a second to consider our choices for a bit longer.”
Concerning future development, the MIT study suggests that improving dataset transparency could rectify the problem. If researchers were aware of how the data was being gathered, then they know how the data should be used. MIT researchers also want to explore transfer learning in future work, which includes fine-tuning a descriptively trained model on a small amount of normative data.
Lead author Marzyeh Ghassemi, who is also assistant professor and head of the Healthy ML Group in the Computer Science and Artificial Intelligence Laboratory (CSAIL), said: “The way to fix this is to transparently acknowledge that if we want to reproduce human judgement, we must only use data that were collected in that setting.”
“Otherwise, we are going to end up with systems that are going to have extremely harsh moderations, much harsher than what humans would do. Humans would see nuance or make another distinction, whereas these models don’t.”