Neural networks help minimise garbage project wasted efforts
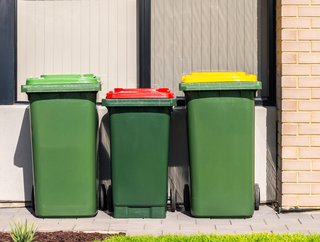
Neural networks can help local authorities forecast which days are going to cause a spike in garbage creation and then schedule waste collection around these predictions, claim researchers.
Sabbir Ahmed, a PhD student at the University of South Australia, is developing a deep-learning model to predict the accumulation of waste in cities and optimise the frequency of public bin clearance. His approach involves leveraging algorithms to analyse data from smart bin sensors.
“Sensors in the public smart bins can give us a lot of information about how busy specific locations are, what type of rubbish is being disposed of and even how much methane gas is being produced from food waste in bins,” says Ahmed.
“All that data can be fed into a neural network model to predict where bins in parks, shopping centres and other public places are likely to fill up quickly and, conversely, which locations are rarely visited. This can help councils to optimise their waste management services, schedule bin clearances and even relocate rarely used bins to where they are needed most.”
Ahmed is collaborating with Wyndham Council in Victoria on a pilot project, using their smart bin data to develop an AI model which local councils across the country could use to make waste services more efficient.
Details of the research were published in the International Journal of Environmental Research and Public Health.
Rapid population growth causes problems
Co-author of the paper, UniSA lecturer Dr Sameera Mubarak, says waste management is a growing concern worldwide. “Many urban areas are struggling to cope with an increase in garbage due to rapid population growth and waste services are becoming increasingly difficult for local governments to manage,” she says.
In developing an AI model, the researchers have analysed sensor data from public bin sites, routing paths and pick-up locations. The sensors capture different types of waste: solid, organic, industry or chemical waste, medical waste, and recycling waste.
“Human planning takes time, but using artificial intelligence, we can predict patterns of waste generation in public sites,” says Dr Mubarak. “This includes forecasting which days are busier in certain locations, flagging upcoming events that will result in a spike in garbage, and then scheduling waste collection around these predictions.
“Improper waste collection can cause serious health and environmental hazards for cities, particularly when bins overflow. This research ticks many boxes, including addressing challenges around sustainability, environmental and health issues, and efficient resourcing.”
The researchers plan to investigate the impact of socioeconomic factors and public utility investment on waste generation in future work.